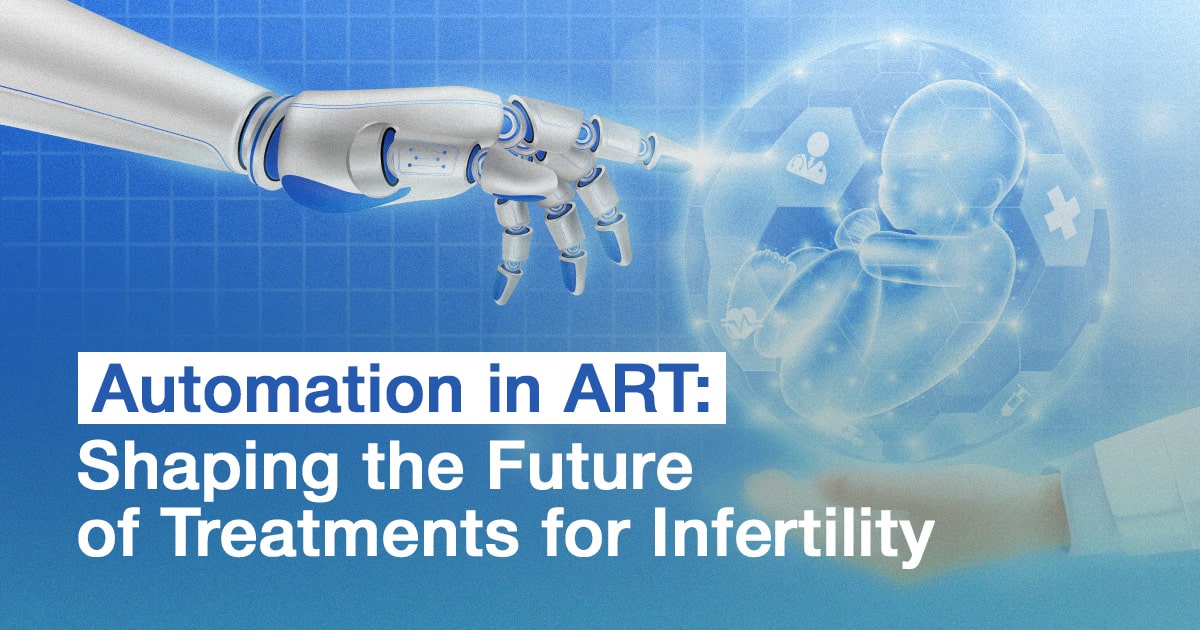
Introduction
In the era of digitalization, automation emerges as a promising solution to enhance efficiency, reproducibility, and consistency in ART. Automation involves the mechanization of tasks traditionally performed by humans or even tasks previously deemed impossible, facilitated by computerized systems. In the medical field, automation is largely realized through robotic systems and microfluidics. Artificial intelligence (AI) complements automation by providing memory through learning and the ability to perform measured actions without direct human interaction. AI is broadly classified into narrow, general, and strong AI based on its capability to handle various tasks. Currently, the world has achieved only narrow AI, the least advanced form. A subset of AI includes machine learning (ML) and natural language processing (NLP), which enable learning from input data and understanding human language, respectively, without explicit programming. The shift from traditional machine learning to deep learning algorithms like artificial neural networks (ANN), convolutional neural networks (CNN), and transformer neural networks (TNN) has been notable. Deep learning utilizes hierarchical representations to analyze extensive datasets with reduced reliance on human involvement. As we anticipate the next wave of biomedical advancements, automation integrated with AI is poised to revolutionize ART success rates. This transformation can be realized by incorporating automation and AI into key steps in ART.
Automated Patient Identification in A.R.T
"To err is human" is a common saying in everyday life, but it is not an acceptable justification for errors within an in vitro fertilization (IVF) laboratory. Although rare and difficult to accept, human errors can occur in the IVF lab, leading to the loss of gametes or, worse, the incorrect implantation of mismatched gametes. The daily tasks of the IVF lab involve intricate procedures with precise multi-step protocols, providing numerous opportunities for errors, especially when handling increasing patient volumes throughout the day.
Automated patient identification has been realized through electronic witnessing systems (EWS) and radio frequency identification (RFID) technology. The electronic witness system (EWS) in in vitro fertilization (IVF) clinical practice is a notable innovation, particularly prevalent in the UK due to specific legislation mandating its use. Despite recommendations to enhance traceability and reduce IVF mix-ups.
Thorough tracking of patient specimens requires monitoring the contents of each dish at every step of the IVF process. National and international organizations, such as the European Society for Human Reproductive and Embryology (ESHRE), ASRM, and HFEA, have proposed best practices emphasizing accurate initial labeling with supervision or a "double witness" to start the process. In addition to double-witnessing protocols, electronic witnessing systems (EWS), such as barcode identification or radiofrequency identification, have been introduced. These commercial systems are based on labeling all labware used for each case with barcode stickers or radio frequency identification labels, identified by unique computer-based readers.
While these systems minimize the risk of sample mismatch due to human error, the possibility of misidentification still exists as gametes and embryos are moved during an ART cycle. To track dish contents, manual tagging of oocytes and embryos with polysilicon barcodes has been proposed. However, this labeling process is invasive, time-consuming, and adds complexity to the IVF workflow. For gamete and embryo witnessing to be widely accepted, it needs to be non-invasive, simple, accurate, and easy to incorporate into any IVF laboratory.
Automation in Embryo Selection
The development of single-step continuous culture media for growing embryos to the blastocyst stage has facilitated the adoption of time-lapse imaging (TLI) incubators in human IVF laboratories. TLI enables continuous automated monitoring of embryo development without exposing them to environmental stress. The TLI culture system comprises continuous undisturbed culture and the monitoring of morphokinetic parameters.
The clinical implementation of TLI has led to a significant evolution in embryo grading, progressing from conventional morphology grading to morphokinetic grading, and subsequently integrating artificial intelligence (AI) algorithms—a step toward automation. Numerous studies exploring the benefits of multi-time point evaluation of morphokinetic parameters have fueled interest in TLI and morphokinetic embryo grading. Presently, embryo selection is based on a combination of morphological and kinetic parameters, often using semi/automated or AI algorithms.
However, TLI systems come with challenges, including their high cost, the need for manual annotation of key parameters by embryologists, and susceptibility to inter-observer variability.
AI has been introduced to mitigate human decision-making in TLI, integrating algorithms to enhance the objectivity of assessments. While these AI algorithms are currently in clinical use, they have limitations, and achieving a 100% implantation prediction potential is deemed impossible. Nevertheless, the integration of AI in embryo selection contributes to an increased probability of successful implantation, representing a noteworthy advancement in assisted reproductive technologies.
Future Of A.R.T
Entering the era of Web 3.0 marks a significant advancement in medical sciences, driven by the integration of computer and biomedical disciplines. The areas explored in this discussion, along with potential extensions from fields such as soft robotics and telesurgery, could bring about a transformative shift in Assisted Reproductive Technology (ART), redefining its processes compared to the current state.
Mixed reality (MR), combining virtual reality (VR) and augmented reality (AR), emerges as a promising avenue for automating patient consultations, a particularly valuable feature in the context of global pandemics. Procedures like transvaginal oocyte retrieval (TVOR) and embryo transfer stand to benefit from MR, offering a notable advantage by overcoming geographical constraints for consultants.
The convergence of microfluidics, artificial intelligence (AI), and robotics holds the potential to realize a fully automated and intelligent device, streamlining the entire IVF treatment pathway.
Conclusion
Integrating AI technology into the realm of IVF clinics may represent the next frontier in advancing personalized reproductive medicine and elevating fertility outcomes for patients. The incidence of infertility, recognized as a global burden of disease, continues to escalate. Additionally, global population projections suggest an impending decline below replacement levels in the near future. The recent global impact of the COVID-19 pandemic has resulted in postponed childbearing and fertility treatments for many individuals. Consequently, there is an anticipated surge in the demand for IVF services in the coming years. Given the stagnation in IVF success rates over the past decade, AI emerges as a promising tool to refine and augment current clinical practices, enhancing the predictive capacity of IVF outcomes. This advancement is poised to benefit patients and contribute to the birth of a healthier generation conceived through IVF.